RT2 lncRNA PCR Array Handbook RT2 lncRNA PreAMP cDNA Synthesis Handbook. Found in: RT2 lncRNA qPCR Assay Handbook. Please read the handbook supplied with the RT2 Profiler PCR Array, paying careful in the miScript miRNA PCR Array Handbook as this will allow the use of the QIAGEN handbooks can be requested from QIAGEN Technical Service.
- Rt2 Profiler Pcr Array Handbook Qiagen
- Qiagen Rt2 Profiler Pcr Array
- Rt2 Pcr
- Rt2 Profiler Array
- Qiagen Rt2 Pcr Array
- Qiagen Rt2 Profiler
- Rt2 Profiler Pcr Array Manual
Associated Data
Below is the link to the electronic supplementary material.
Abstract
Microarray technology allows us to perform high-throughput screening of changes in gene expression. The outcome of microarray experiments largely depends on the applied analysis methods and cut-off values chosen. Results are often required to be verified using a more sensitive detection technique, such as quantitative real-time PCR (qPCR or RT-PCR). Throughout the years, this technique has become a de facto golden standard. Individual qPCRs are time-consuming, but the technology to perform high-throughput qPCR reactions has become available through PCR-arrays that allow up to 384 PCR reactions simultaneously. Our current aim was to investigate the usability of a RT2 Profiler™ PCR-array as validation in a nutritional intervention study, where the measured changes in gene expression were low. For some differentially expressed genes, the PCR-array confirmed the microarray prediction, though not for all. Furthermore, the PCR-array allowed picking up the expression of genes that were not measurable on the microarray platform but also vice versa. We conclude that both techniques have their own (dis)advantages and specificities, and for less pronounced changes using both technologies may be useful as complementation rather than validation.
Electronic supplementary material
The online version of this article (doi:10.1007/s12263-008-0094-1) contains supplementary material, which is available to authorized users.
Introduction
Gene expression microarrays have become the leading technology to evaluate changes in genome wide gene expression under different conditions. To confirm results from microarray studies, a selection of differentially expressed genes of interest is generally verified using a highly sensitive technique, mostly a quantitative real-time reverse transcriptase PCR (RT-PCR/qPCR) reaction [, ]. Even though qPCR is considered to be a “golden standard” or a necessary validation [, , , , ], it has also been stated that qPCR results are not always better [] or validation is not always necessary []. In a qPCR reaction, cDNA is synthesised from mRNA molecules present in the sample, which is then amplified exponentially using a combination of gene-specific primers, labelled nucleotides and the DNA polymerase enzyme, theoretically doubling the amount of cDNA at every cycle. Upon analysis, the expression level of the target gene is computed relative to the expression level of one or more reference genes, often housekeeping genes. Selecting proper housekeeping genes is one of the most critical aspects of the analysis, since they need to be constantly expressed between all samples and conditions in the experiment. A survey of publications reveals a wide range in correlations between microarray and qPCR results [–, , ].
To allow qPCR in a more high-throughput way, several companies have developed a practical solution to perform up to 384 qPCR reactions on one single plate, such as the TaqMan™ Gene Expression Arrays from Applied Biosystems and RT2 Profiler™ PCR Array System from SABiosciences. PCR-arrays have mainly been used in cancer research, but also in some other fields, for example, drug metabolism []. At the time of writing, 74 RT2 Profiler™ PCR-arrays are catalogued that contain primer sequences for genes belonging to a specific function, structure or pathway (e.g., apoptosis, common cytokines, NF-κB signalling pathway) or disease (e.g., cancer). In addition, arrays can be custom-made. For the 96-well system, every plate contains five housekeeping genes (B2M, HPRT1, RPL13a, GADPH and ACTB), three positive PCR controls, three reverse transcriptase controls and one control to detect the presence of genomic cDNA contamination. Correlations between results of several RT2 Profiler PCR-arrays and qPCR have been reported to be good [, ].
In human nutritional intervention studies, the response of a given diet on gene expression can be minimal. Most publications in this field make use of qPCR to validate a few genes of interest [, ], but no larger scale approaches have been used. The goal of this study is to evaluate if for this purpose a PCR-array can be used in a dietary intervention study where the expected changes are small.
Materials and methods
This paper elaborates on a study investigating the relationship between diet and inflammation []. For microarray analysis, mRNAs from peripheral mononuclear blood cell (PBMC) samples of eight obese subjects were collected. These subjects participated in both the fish oil (FishOil) and weight loss (WeightLoss) interventions. More details about the study design are provided in the original paper [].
RNA was pooled with the control (Cy3) being the control diet and with either the WeightLoss or FishOil end-point as Cy5. RNA quantity and purity were determined using the NanoDrop ND-2000 (Nanodrop Technologies, Wilmington, DE, USA), and RNA integrity was assessed by determining the RNA 28S/18S ratio using the Bioanalyzer 2100 (Agilent Technologies, Santa Clara, CA, USA). Pooled RNA samples were hybridised to Agilent Human 1A G4110B microarrays, containing 22,575 reporters. This was done according to a dye-flip design, resulting in a total number of four arrays. Array quality control was performed in Spotfire DecisionSite 9.0 and R 2.5.1 using the limma library version 2.10.5 [17]. Reporters passed quality control when (1) signals of both channels consisted of more than 55 pixels; (2) both signals were higher than 2.6 times the standard deviation of the local background and (3) at least one signal was not saturated. All further data analyses were performed in R. Within-array normalisation was performed by using the LOESS algorithm. Next, a linear model was fitted for every reporter and a moderated t test was performed. To ensure microarray reporter specificity, all reporters were reannotated using stable UniProt and EnsEMBL identifiers [].
Microarray results were validated using a specific PCR-array (SABiosciences, Frederick, USA) that met two criteria. We selected all human PCR-arrays that contained a relatively large number of genes that were differentially expressed (P value < 0.05 and |FC| > 1.2) by the two treatment comparisons performed in the microarray study and that contained a gene set that was related to the observed changes in plasma metabolic parameters. Based on the outcome, the 96-well plate Human Common Cytokines PCR-array (PAHS-021) was selected.
The mRNA of each individual was converted into cDNA using the RT2 First Strand Kit (SABiosciences, Frederick, USA). This cDNA was then added to the RT2 SYBR Green qPCR Master Mix (SABiosciences, Frederick, USA). Next, each sample was aliquotted on 24 Human Common Cytokine PCR-arrays. All steps were done according to the manufacturer’s protocol for the ABI Prism 7000 Sequence Detection System. RNA quality was good, with 260/280 ratios slightly higher than 2.0 and 260/230 ratios slightly higher than 1.8. To analyze the PCR-array data, an MS-Excel sheet with macros was downloaded from the manufacturer’s website (http://www.sabiosciences.com/pcrarraydataanalysis.php). The website also allowed online analysis. We preferred to use offline calculations since it was not possible to save the results online, and even more importantly because it was easier to track the formulas and to understand what was being done. For each PCR reaction, the Excel sheet calculated two normalised average Ct values, a paired t test P value and a fold change. To filter out undetectable genes, an extra quality check was added by us: for each intervention, the PCR gene product was flagged as either “good” or “bad”. A gene was considered good when in both the control and the dietary intervention the difference between the measured genomic cDNA Ct value (maximum 35) and the measured Ct value of the gene was at least three cycles in the same five out of eight subjects for each comparison. For each included gene, individual measurements that were not detected were excluded from further analysis. This was done to correct the lack of robustness of the analysis spreadsheet, as provided by the manufacturer, and to take into consideration the outlying values that have a considerable influence on the calculated fold change and its associated P value. Data normalisation was based on correcting all Ct values for the average Ct values of several constantly expressed housekeeping genes (HKGs) present on the array. PCR-array analysis results were evaluated and also compared to the outcome of the gene expression microarrays.
Results and discussion
In our current analysis, only four HKGs (HPRT1, RPL13A, GAPDH and ACTB) were used for normalisation. Beta-2-microglobulin (B2M) was not included, since it was significantly altered on the PCR-array after the weight loss period. Applying the flagging criteria resulted in finding 29 and 33 detectable genes after the FishOil and the WeightLoss intervention, respectively. All detected genes in the FishOil intervention were also measured accurately in the WeightLoss comparison. The flagging procedure removed several observations that were most likely caused by failing amplifications, since only one value of one subject was undetectable (Ct > 35). Note however that in some cases this flagging may be somewhat conservative: for example, for two subjects in the FishOil experiment, the IFNK gene changed from baseline undetected (Ct = 35) to Ct values of 30.27 and 30.72 cycles. All other subjects had a nearly constant Ct value ranging between 29.5 and 29.8 cycles. The same pattern occurred for two subjects, and therefore it could well be a real biological effect. In general, the measurements obtained by the PCR-array were consistent between subjects and show very small variation, as in regular qPCR.
Table 1 summarizes our findings with respect to the detectable genes on the PCR-array and their corresponding microarray values. The coverage of the detected PCR genes on the reannotated microarray was 100%. Seven PCR genes were present more than once on the Agilent microarray platform. For two of those genes (FASLG and TNF) their corresponding reporters on the microarray were identical, specific, and showed only a slight difference in fold change. The other five genes (ACTB, CSF1, PDGFA, TFGA and TFGB1) had two different microarray reporter sequences that align to different parts of the gene product. For these seven genes, the fold change for the highest expressed reporter was chosen to represent the gene on the microarray. It was not possible to measure the expression of five of the PCR-measured genes on the microarray: two genes (B2M, TNFSF14) were always saturated, whereas the other three (BMP8B, CSF1, IFNK) were filtered out during microarray quality control due to a too low background-corrected intensity value. All other genes on the PCR-array, apart from IFNA1, were also present on the reannotated microarray, where IFNA4 was only covered by reporters targeting several of its family members as well. Within the complete list of genes (cf. Supplementary Table 1), only ~10% of genes detected on the PCR-array could not be detected on the microarray. Conversely, ~50% of genes, for which primers were available on the PCR-arrays, could be detected on the microarray but not on the PCR-array. This result contradicts the original hypothesis of a better sensitivity of qPCR in comparison to microarray [, ]. This could be explained by the different criteria used and may point to the fact that a required threefold difference in Ct value compared to the genomic control, as suggested by the manufacturer, is too strict. However, this cannot offer an explanation for all the findings, illustrated by the INHA gene, which was detectable on the microarray in low concentrations and significant for all comparisons, but had Ct values of ~35 on the PCR-array. On the other hand, with highly expressed genes, the microarray measurements were sometimes saturated. This saturation did not occur on the qPCR. Thus, the reported lower dynamic range of microarray measurements [, ] was confirmed for this end of the spectrum. In principle, a simple rescanning of the array at a lower scanning intensity could overcome this problem. However, such rescanning procedures have to be done shortly after hybridisation, and in our experience, in practice, this is not often done. We did not observe that array performance would be especially lower with lower expression levels or fold changes, as reported before [, –, , ], though several authors also found acceptable detection levels of lower fold changes or expression levels or less correlation between expression and accuracy [, , ]. In our study, consistency in direction of change between both methods remained high down to the 10% change range for the qPCR. Finally, even though a trend of the microarray in estimating smaller fold changes as compared to qPCR was observed [, ], the difference was quite limited.
Table 1
Detectable reporters on RT2 Profiler™ PCR-array in human PBMCs
Gene | Number of reporters on MA | FishOil versus Control | WeightLoss versus Control | ||
---|---|---|---|---|---|
FC–MA | FC–PCR | FC–MA | FC–PCR | ||
ACTB | 2 | 1.01 | −1.05 | 1.03 | −1.06 |
B2M | 1 | N/A | −1.09 | N/A | −1.23* |
BMP6 | 1 | −1.02 | −1.39 | −1.17* | −1.15 |
BMP8B | 1 | N/A | −1.42 | N/A | 1.11 |
CSF1 | 4 | 1.11 | 1.04 | −1.05 | −1.15 |
FASLG | 2 | −1.01 | −1.11 | −1.04 | −1.04 |
GAPDH | 1 | −1.01 | −1.08 | −1.07 | −1.06 |
GDF11 | 1 | −1.02 | −1.09 | −1.07 | 1.05 |
HPRT1 | 1 | −1.00 | −1.11 | −1.11* | −1.05 |
IFNG | 1 | −1.03 | −1.06 | −1.21* | −1.07 |
IFNK | 1 | N/A | N/A | N/A | −1.38 |
IL15 | 1 | −1.03 | −1.21 | −1.08 | −1.05 |
IL16 | 1 | 1.03 | −1.07 | −1.13* | −1.08 |
IL18 | 1 | −1.10 | −1.13 | 1.02 | −1.07 |
IL1B | 1 | 1.05 | −1.06 | 1.03 | 1.02 |
IL24 | 1 | 1.20* | −1.14 | −1.29* | 1.05 |
IL7 | 1 | N/A | N/A | −1.19* | −1.43 |
LTA | 1 | 1.01 | 1.05 | −1.09* | −1.09 |
LTB | 1 | 1.00 | −1.05 | −1.06* | −1.12 |
PDGFA | 2 | −1.10* | −1.24 | −1.36* | −1.46 |
RPL13A | 1 | 1.02 | −1.01 | −1.05 | −1.08 |
TGFA | 2 | −1.03 | 1.03 | −1.15* | −1.07 |
TGFB1 | 2 | 1.07 | −1.03 | −1.01 | 1.02 |
TGFB3 | 1 | N/A | N/A | 1.06 | 1.32 |
TNF | 2 | 1.09* | −1.10 | −1.07 | −1.12 |
TNFSF10 | 1 | −1.07* | −1.14 | 1.10* | −1.18 |
TNFSF12 | 1 | 1.03 | −1.06 | −1.03 | 1.03 |
TNFSF13 | 1 | −1.02 | −1.03 | 1.00 | −1.01 |
TNFSF13B | 1 | −1.08 | −1.23 | −1.00 | −1.03 |
TNFSF14 | 1 | N/A | −1.33 | N/A | 1.02 |
TNFSF4 | 1 | N/A | N/A | −1.28* | −1.48 |
TNFSF8 | 1 | −1.08* | −1.25 | 1.01 | 1.02 |
TXLNA | 1 | −1.13* | 1.08 | 1.07* | −1.06 |
Calculated fold changes of 34 detectable PCR gene products as compared to the fold changes derived from the microarray experiment for each intervention
FC fold change, MA microarray, N/A not available, PCR PCR-array
* P < 0.05
With respect to significantly regulated genes, some changes were consistent while few were not (cf. Table 1 and Supplementary Table 1). The P values of the PCR-array were much higher than the P values of the microarray. However, the microarray material was pooled, whereas the PCR samples were not, resulting in much lower variation for the first analysis technique. Since this would logically lead to lower P values, this effect was not taken into account for the comparison. The majority of genes on both the PCR-array and the microarray were not differentially expressed for either technology. Among differentially expressed genes the PDFGA gene was detected as significant under both experimental conditions on the microarray and its computed fold changes were confirmed on the PCR-array. For TNFSF4, the results also corresponded: for both platforms downregulated for WeightLoss and flagged for FishOil. TNFSF8 showed slight downregulation for FishOil but no change for WeightLoss. Other genes were less concordant with IL24 as most conflicting gene, showing opposite values for both comparisons. Bad annotation or crosshybridisation are unlikely causes for this inconsistency, since both arrays were very carefully annotated in our study, as the microarray contains specific probes for this gene, and the PCR primers were designed to be specific for all gene variants. It may however be explained by a possible difference in splice variants detected by the two technologies, as IL24 has more than one associated transcript. It is known that between-platform consistency decreases with different primers [, , ] or increased distance on the sequence between them []. Unfortunately, since the primer sequences of the PCR products are not publicly available, we cannot verify this possible cause of discrepancy.
In conclusion, we have observed consistencies and differences between results obtained by two different technologies. In general, we could not observe a better detection level of the PCR-array, though this may be caused by the chosen quality criteria set. Especially with different probes/primers, it is hard to decide which technology gives a more accurate measurement. Different results may be considered complementary instead of contradictory. Especially, for nutritional studies, where effects can be expected to be small, one could argue that running a microarray with a broader coverage could be followed by using a PCR-array focused at a specific process, to possibly detect extra transcripts. However, current and future costs will also play a role in the choice of the platforms to be utilized. Finally, we conclude that PCR-arrays should not be considered a perfect validation tool, since both array technologies have their own specific detection limits and neither can be considered to be the real golden standard.

Electronic supplementary material
Below is the link to the electronic supplementary material.
Acknowledgments
This research was partly funded by the Nutrigenomics Consortium of the Top Institute Food and Nutrition (TIFN), the Dutch BSIK Fund and NuGO. The authors would like to express their thanks to M. Hulsbosch for performing the PCR-array experiments. We also thank the support staff at Tebu-Bio Netherlands for their helpful suggestions during the data analysis.
Conflict of interest statement The authors declare no conflicts of interest.
Rt2 Profiler Pcr Array Handbook Qiagen
Open Access This article is distributed under the terms of the Creative Commons Attribution Noncommercial License which permits any noncommercial use, distribution, and reproduction in any medium, provided the original author(s) and source are credited.
Footnotes
Electronic supplementary material
The online version of this article (doi:10.1007/s12263-008-0094-1) contains supplementary material, which is available to authorized users.
References
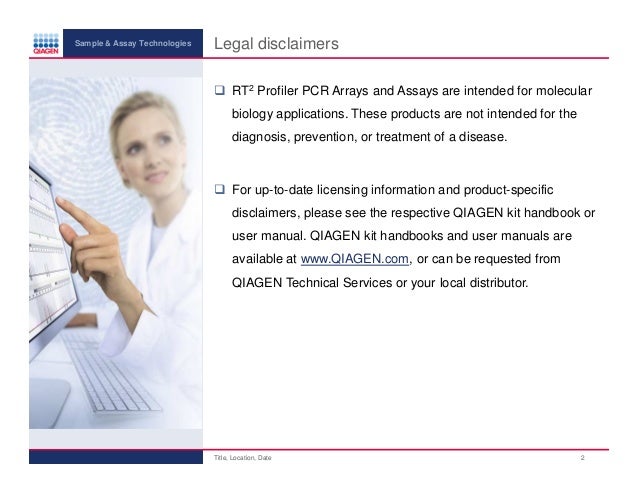
Qiagen Rt2 Profiler Pcr Array
Rt2 Pcr
Rt2 Profiler Array
Qiagen Rt2 Pcr Array
Qiagen Rt2 Profiler
Rt2 Profiler Pcr Array Manual
